Robust Intelligence Releases the AI Risk Database to Evaluate Supply Chain Risk in Open Source Models
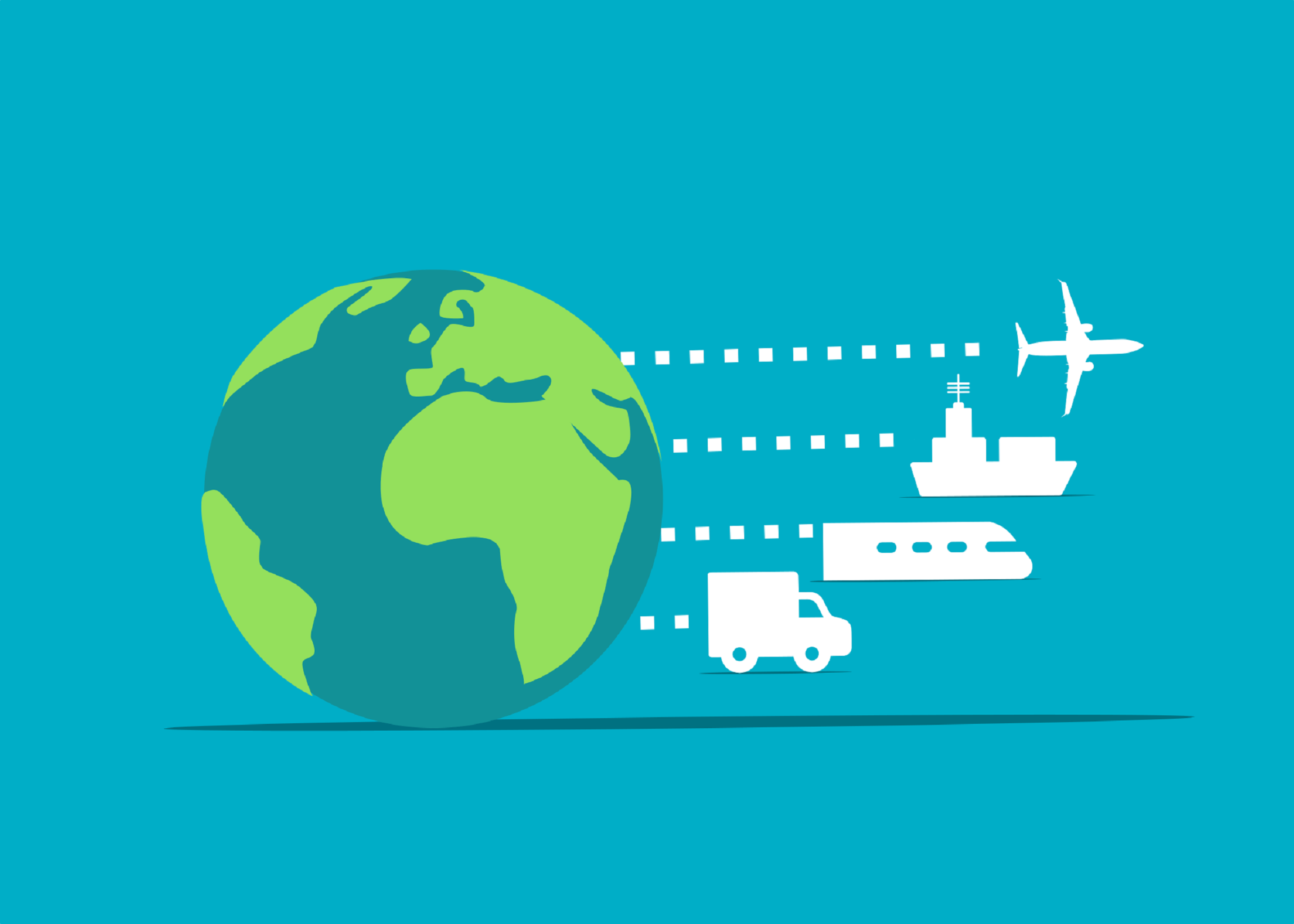
The database is free, community-supported resource that helps protect against AI risk of third-party models
Robust Intelligence, the end-to-end AI integrity company that proactively mitigates model failure, has released the AI Risk Database, a free and community-supported resource to evaluate AI supply chain risk in open source models. The database includes comprehensive test results and corresponding risk scores for over 170,000 models, as well as a growing number of vulnerability reports submitted by AI and cybersecurity researchers.
The rapid pace AI development is driven in part by the availability of public model repositories, including Hugging Face, spaCy, PyTorch Hub, TensorFlow Hub, NVIDIA NGC AI software hub, Papers With Code, and others. These repositories have made sophisticated models widely accessible to organizations; however, it’s incredibly difficult to assess any given model for security, ethical, and operational risks. Statements about model robustness and performance documented in public repos may be unsubstantiated, and many malicious and inadvertent security vulnerabilities are embedded within model resource files themselves. When users download open source models and put them into production, they expose their organization and end-users to considerable risk.
The AI Risk Database makes it simple to understand the security, ethical, operational, and overall health of third-party models and serves as a centralized resource that covers the majority of public model repositories. This enables AI developers to easily evaluate and investigate various models before use and AI researchers to formally report risks they identify. The landing page includes a leaderboard to recognize the developers popular open source models that have the fewest known risks, and another leaderboard to recognize contributors who have submitted the highest-rated reports.
“While public model repositories are accelerating the pace of open source AI development, it’s essential that such models are thoroughly evaluated for risk before use – just as with any public software source. The AI Risk Database and other tools that collate model risks are essential to validate the security and robustness of open source models,” said Beat Buesser, Voting Member of the Technical Advisory Committee of the Linux Foundation AI & Data (LFAI) and maintainer of the Adversarial Robustness Toolbox (ART). “I am pleased by the comprehensive analysis of the AI Risk Database and the inclusion of additional community-supported resources, such as LFAI’s ART to measure model sensitivity to adversarial attacks.”
Read More: SalesTechStar Interview with Ryan Neu, co-founder and CEO at Vendr
The elements of the AI Risk Database
The AI Risk Database offers validation of open source models that is objectively independent from any of the public model repositories. It indexes models from public repositories and summarizes the results from hundreds of automated tests, supplemented by model vulnerability reports. Key elements of the AI Risk Database include:
- a comprehensive database of open source models that is searchable by name or characteristic,
- risk scores derived from dynamic analysis of the models for security, ethical, and operational risks,
- model vulnerability reports from both automated scanners and human contributors,
- a software bill of materials for public models that includes observability into shared resource files across different model repositories, and
- results of scanning model file resources for file-based anomalies (e.g., arbitrary code execution in pickle, pytorch, yaml, tensorflow, and other file formats).
Read More: 5 Ways Data-Driven BDR Leaders Motivate Their Teams
Initial findings reveal significant vulnerabilities
Initial analysis of the automated test results conducted by the AI Risk Database reveal vulnerabilities of varying severity in tens of thousands of open source models. To illustrate how widespread the vulnerabilities are, 50% of public image classifier models tested fail 40% of adversarial attacks and 50% of public natural language processing (NLP) models tested fail 14% of adversarial text transformations. The analysis also revealed dozens of repositories with pytorch, tensorflow, pickle, or yaml resource files that include unsafe or vulnerable dependencies, which at minimum can make the user (and by extension their organization) susceptible to known vulnerabilities and in more severe cases enable actions including arbitrary code execution.
“Open source tools have made AI advancements widely accessible, but the vulnerabilities have created a significant unmanaged risk,” explained Yaron Singer, CEO and co-founder of Robust Intelligence. “Organizations of all sizes are experimenting with, or deploying, models from public model repositories. The AI Risk Database enables the safe use of third-party models, and is one of many steps companies can take to instill integrity in their AI systems.”