Enterprises Seek to Accelerate Data Transformation – Why is it So Difficult?
By Aaron Segesman, Solution Architect at Matillion
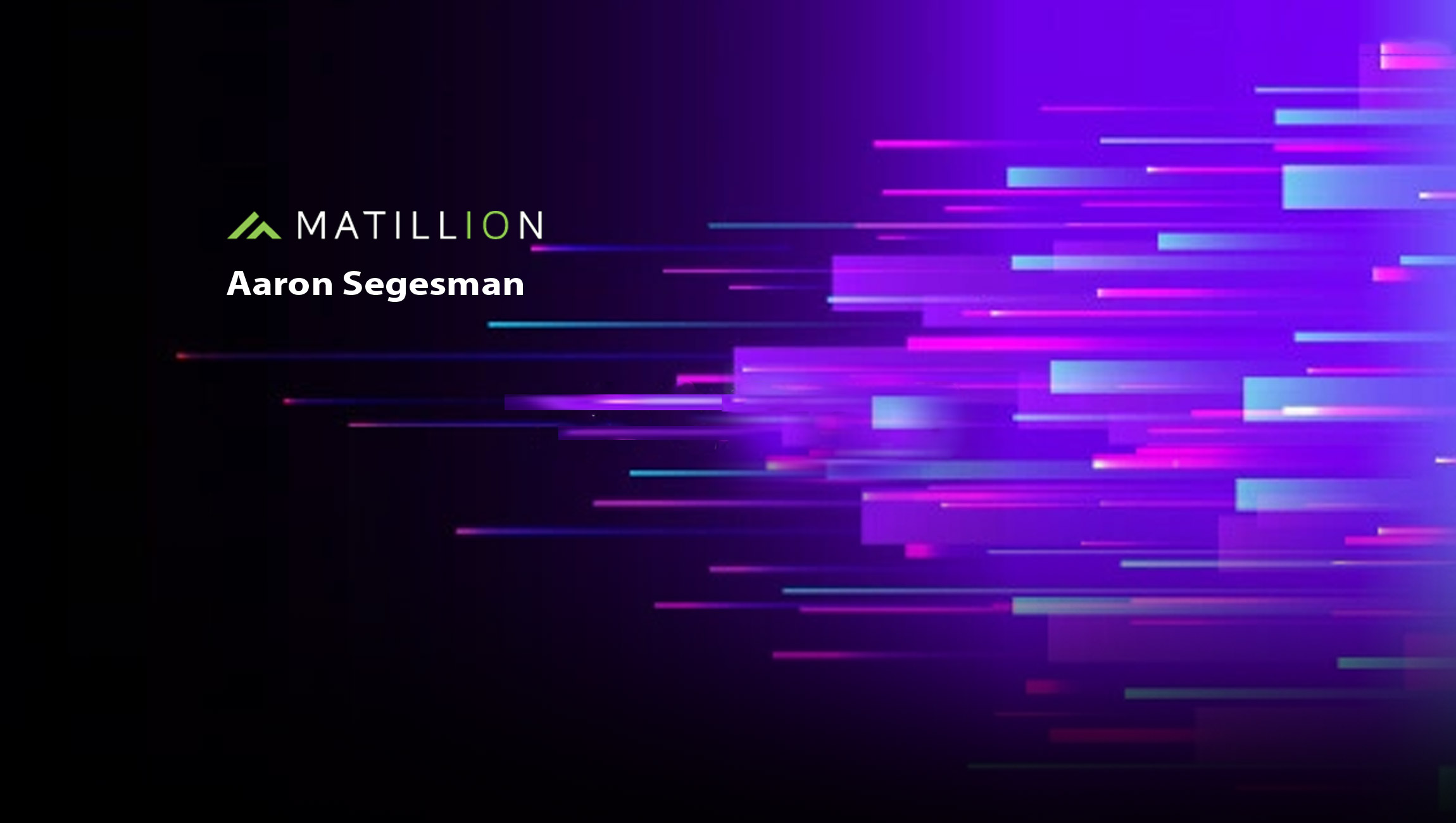
In 2019, data volumes were growing at an average rate of 63% per month, and organizations were coordinating data from as many as 400 different data sources. A recent survey by IDG Research on enterprise data professionals revealed some interesting insights about the amount of data in the world and how enterprise companies are handling it. For example, 97% of enterprises want to accelerate data transformation, but the majority of businesses spend too much time on preparing those immense data volumes from diverse data sources, hindering their ability for insights-driven decision making.
Read More: SalesTechStar Interview With Steve Bryerton, Vice President, Sales At ZoomInfo
There is a clear struggle for enterprises to perform data transformation faster to enable access to analytics-ready insights. Here are some of the challenges that businesses face in accelerating data transformation, and the top requirements needed to help them address these challenges.
Businesses waste too much time wrangling and preparing data
High-quality insights require high-quality data. Unfortunately, for many data teams, high-quality data requires a lot of labor. Simply preparing data for analytics accounts for half of their time, which could be better spent on strategic, high-value tasks that drive the business. Lack of scalability and flexibility are also challenges for enterprises when preparing data for analytics.
Data teams spend an average of one week preparing data for the typical analytics project – that’s not counting the data analysis, reporting findings, preparing dashboards, or any of the other tasks involved with sharing insight across the business. When today’s business climate demands information as closely to real time as we can get, waiting for results can be detrimental to any organization. Speeding up that process can lead to a competitive advantage.
Operational and technical challenges are widespread, making it difficult for enterprises to get data analytics projects to production
Data portability and ease of onboarding are both key in overcoming data analytics obstacles. Nearly half of enterprises report that data control issues are the biggest challenge to data analytics projects. This top challenge is closely followed by lack of a scalable, reliable technology platform available to process large data sets, having too many manual processes, and challenges cleansing and preparing data. Lack of visibility and control of data silos are also a barrier to collaboration with business users on analytics needs.
Blending cloud data platforms presents new opportunities
Enterprises are not thinking about data warehouses and data lakes as an either/or proposition. While more than one-third of enterprises already use cloud data warehouses (CDWs), according to the survey 43% expect to have all of their data in the cloud and the remainder planning to pursue hybrid models that leverage both cloud and on-premises data warehouses. Use of CDWs is already widespread – some using data lakes to store both structured and semi-structured data, with many others planning to use data lakes in the future. Likewise, the majority will leverage a hybrid cloud strategy (on-premises and cloud) for data management. Even more are planning a multi-cloud strategy. This demand for both types of cloud environments makes clear the opportunity for technologies like the lakehouse, which leverages the features of both the cloud data warehouse and the data lake for a myriad of use cases.
Read More: National Tree Company Revamps Order Processing With Hewlett Packard Enterprise Just In Time For The…
Data portability, time-to-value, and self-service are critical for business users to overcome challenges
Data portability, ease of onboarding, and cost effectiveness are the top features of an analytics platform that can help enterprises move past current obstacles. Data portability is crucial because data teams regularly need to rely on the same data across multiple environments: on-premises, cloud, and increasingly, across different cloud platforms.
IT professionals favor user-friendly and easier connections to data sources as top features, while data professionals (data engineers, data scientists, data architects, etc) favor time-to-value and the ability to provide self-service as important capabilities.
Ease of onboarding and use of a product is also a critical quality because multiple users can increase data team agility and productivity. A full range of users across the business–data engineers, data analysts and data scientists, as well as business data users–can help balance workloads and democratize data.
Governance and data accessibility are on everyone’s mind
Data democratization is the eventual goal of many modern organizations, making data available to different groups to self-serve analytics. However, the biggest obstacles to getting data analytics projects over the finish line continues to be data ownership and data control issues that make collaboration difficult. Organizations continue to realize that establishing governance and data stewardship will lift a significant barrier to analytics productivity and overall data quality.
Look to cloud-native solutions to move faster with data
There are challenges that are holding enterprises back, but more importantly, there are capabilities and features they can leverage from a strategy to deliver business value through transformed data. The power and the economics of cloud-native solutions help companies achieve faster time to value that has a big impact in creating a data-driven culture for IT and business users.
Read More: SalesTechStar Interview With Steve Bryerton, Vice President, Sales At ZoomInfo
———
What’s New on The SalesStar Podcast? Listen Now!