Data Is the Differentiator for Best of Breed Sales Readiness Platforms
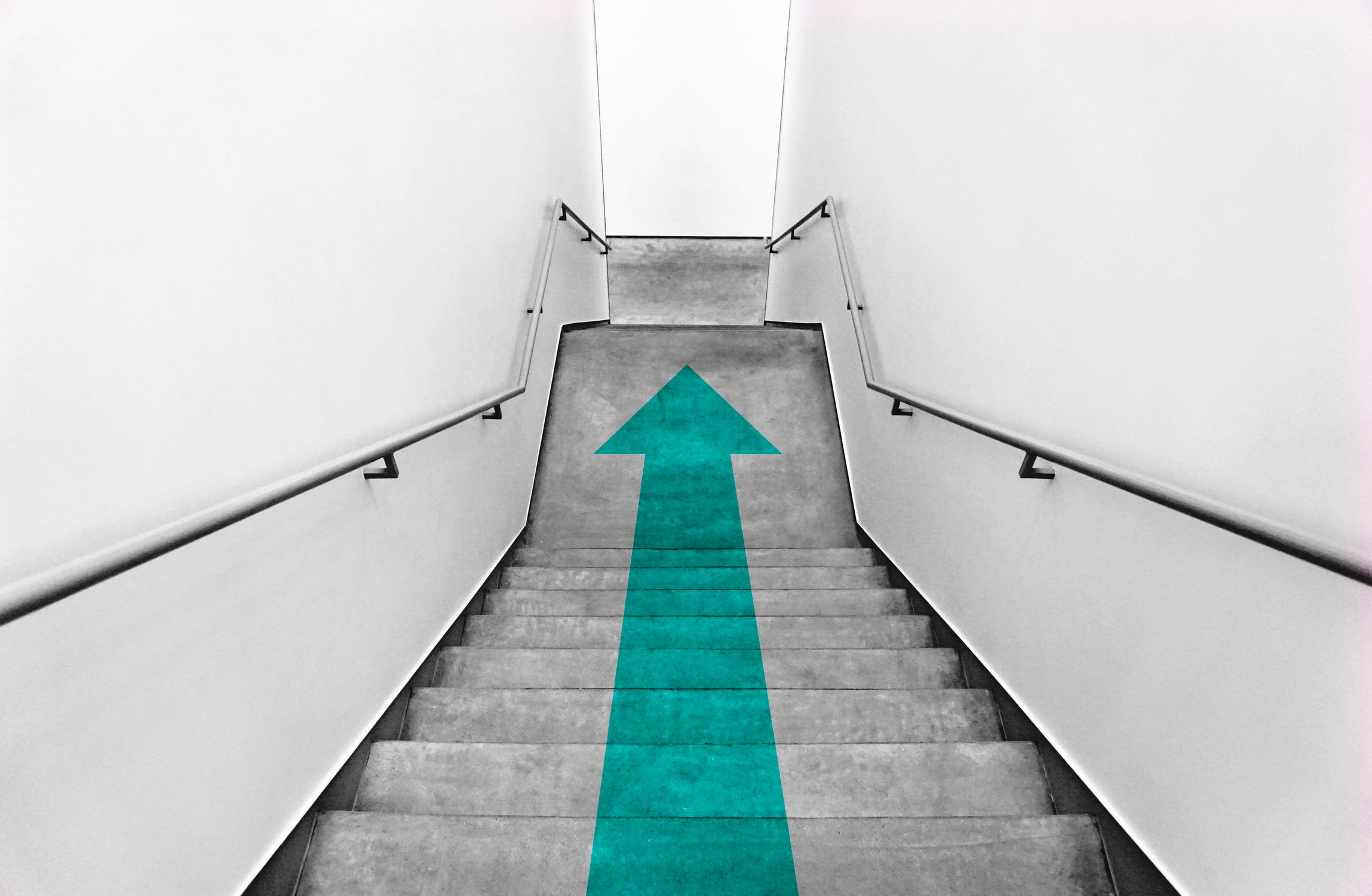
Nearly a decade ago, sales leaders realized they had a problem with sales training. Then there was a rush to figure out how technology could improve sales training. This led to the current generation of sales training software which aimed to improve user engagement and knowledge retention through cloud-based, on-demand content and more seamless experience whether delivered via mobile or desktop. They broke down the monolithic learning experience using elements of gamified micro-learning and mechanisms that made learning content available anytime and anywhere. In their current incarnation, these new solutions continue to displace legacy sales training systems as a better choice for sales, customer service, or any revenue generating employee.
Because of the mobile-first and SaaS approach, the current generation of the sales training solutions has an opportunity to capture every user interaction and more importantly, the context around that interaction over their entire customer base and across verticals. Capturing these interactions, however, leads to a huge volume and variety of structured and unstructured engagement data that includes scores, completions, clicks, text, audio, video and more.
Organizing and deriving value from that data add an additional layer of complexity which demands an extremely sophisticated data management architecture. An architecture which the majority of the current generation of sales training solutions simply lack.
A best of breed sales readiness platform should be able to convert this engagement data into a common data model and organize the sellers, content, and programs into cohorts based on metrics derived from the raw engagement data. These derived metrics serve as the optimal leading indicators of seller capabilities which, in turn, are much better correlators to business outcomes than simple scores and completions. In short, the engagement data holds the key to unlock the answer to the ultimate question –
How do I maximize my readiness investments and make my sales person ready?
There are a few aspects of how a platform should leverage data to create a hyper-personalized experience and drive differentiation from the rest of the sales training solutions.
Building a Sales readiness intelligence data store
The ultimate goal behind creating a data store is finding competencies that best correlate with sales’ performance and activity metrics, and ultimately optimize the readiness investments to develop those competencies. However, this level of visibility requires the readiness solution to have the ability to tag various programs and content created within it by their business goals, and the skills and competencies needed to attain them. At any moment, a sales manager should be able to readily answer questions like:
- What are the current strengths and weaknesses of my team around the competencies they need to have?
- How does my team compare to other teams?
- Is my team improving over time?
For Sales enablement leaders, a natural next step after understanding the current status of core competencies is figuring out how to best ensure that readiness investments are optimized around these competencies so they can prevent the competency gaps from developing. This requires access to data that can uncover the leading indicators of competency development.
In order to present these leading indicators, an optimal readiness solution needs to collect raw data around content metadata, engagement, activity, content, audio, and video from practice or live calls, audio-to-text transcripts, review feedback, and comments all in one store. And to begin to make sense of this data, a level of preprocessing and aggregation built into the data store is required to derive important higher order metrics from the raw data.
Examples of these kinds of metrics are:
- Competency proficiency
- Program structure quality
- Content effectiveness
- Seller proactivity
- Seller confidence
- Seller activity level
Ensuring the data is ‘complete’
Interestingly, partial data is more harmful than no data at all because data completeness is a function of breadth, history, and accuracy. Without data breadth, it’s difficult to pinpoint the exact nature of a problem. For example, it’s challenging to tell things like whether a competency gap stems from a lack of knowledge, skills, execution, or all of the above. You can ensure data breadth by collecting data across the complete lifecycle of knowledge development (based on micro-learning completion), skill development (role-plays) and behavior (live coaching and field observation, or analysis of real customer calls).
Ensuring data history and accuracy requires a level of rigor and coordination between the product feature teams and the data engineering teams. Both must ensure that any relevant user interaction is utilized and optimized to best capture the data and route to a central data store – a practice that many organizations struggle with keeping up.
The need for complete data to understand sales competencies makes it imperative to ensure that you don’t rely solely on point solutions, but implement an end-to-end readiness platform. Often, vendors liberally use the word ‘platform’ as an umbrella term for any readiness solution. The reality is, however, that most are limited, point solutions for use cases like sales coaching or micro-learning or conversational intelligence. While they might be enough for the respective use cases, it becomes tough to effectively integrate the data sets from all the disparate point solutions. In these cases, all of the data ends up scattered across multiple vendor databases and stored in varying formats under different taxonomies.
Creating and leveraging the data network effect
Frequently, when customers consult with us on how they could make better use of our data to improve their programs, I’ve been asked to share examples regarding how other customers tackle it. Since enablement as a function is still maturing, there’s significant room for the community to grow and learn. Avenues like the Sales Enablement Society create a medium for cross-pollination and sharing of ideas, but those interactions don’t happen frequently enough. Since the more robust readiness solutions come across examples of good and bad implementations across verticals and use cases, they should find the time to share those insights across their customer base. That way, customers can learn from each other and benefit from being part of the same platform.
For example, when a customer asks about how to structure their onboarding program to maximize engagement and knowledge retention, an ideal solution should come back with a few prescriptive recommendations built into the platform itself. These can range from how to optimally interleave questions within the content, to structuring content around competencies, to optimizing the timing of invitations and reminders.
Personalizing data-driven insights
Data is only useful when the derived insights are presented in a consumable manner to the various personas interacting with the solution. While indeed, most readiness solutions in the market support some form of reporting – it is the bare minimum. The conversation between enablement and sales management, particularly when it comes to reporting, needs to shift from being solely focused around completions of specific programs to core competencies.
A sales manager is much more likely to pay attention if you tell them that their team is expected to be in the lowest performing quartile than, for example, merely asking them to ensure that their team has completed all their ‘assigned’ courses and missions.
This kind of conversation requires a level of personalization that makes reports extremely relevant to specific reps and managers based on their individual skills and knowledge gaps. It also requires the ability to upload company-specific and role-specific data from various systems like CRM, HR, and identity management to accommodate specific situations.
Lastly, to motivate actionable steps around these insights, capabilities like the ability for admin to bookmark reports for managers or schedule delivery by email are key.
Surface the Insights ‘In-Context’ to drive recommendations
Personalizing the insights and making them focused around competencies is an essential first step. Moreso, if these insights are used in the right context, they can help power even more contextual recommendations. For example, when the sales manager is reviewing a role play, they can be given some in-context pointers as to the rep’s current pain points. Alternatively, they can receive a notification that shows them the exact next steps when it comes to specific micro learning or role play assignments already available in the system to which they can direct their reps.
Similarly, when a seller logs in to complete a course or complete a quiz question, they should be able to see how well they’re doing within their peer group. Motivational information can be provided on how many points they need to progress to the next level. It’s through these subtle and contextual applications of the insights that seller engagement and competency development can be optimized.
Feed ML/AI Algorithms
With readily available open source and cloud-based machine learning platforms – as well as APIs – today’s barrier to AI/ML adoption is pretty low. In fact, you’ll be hard pressed to find a training or enablement solution today that doesn’t tout its use of ML/AI. That said, these algorithms are only as good as the data being used to teach them. Further, these algorithms produce a much higher quality output if, instead of being trained using simple raw data, they are fed the derived metrics.
So, while the sales training space has benefitted from some innovations like being SaaS and cloud-based only when a new data architecture is developed can the real value be unlocked with the ability to store, process, and analyze an incredible wealth of data, especially in a SaaS-based model. And only when a best of breed readiness solution can combine this with business outcome data and leverage machine learning will we see the true potential to surface insights, drive personalized recommendations and optimize sales performance.