Is AI in Sales DOA?
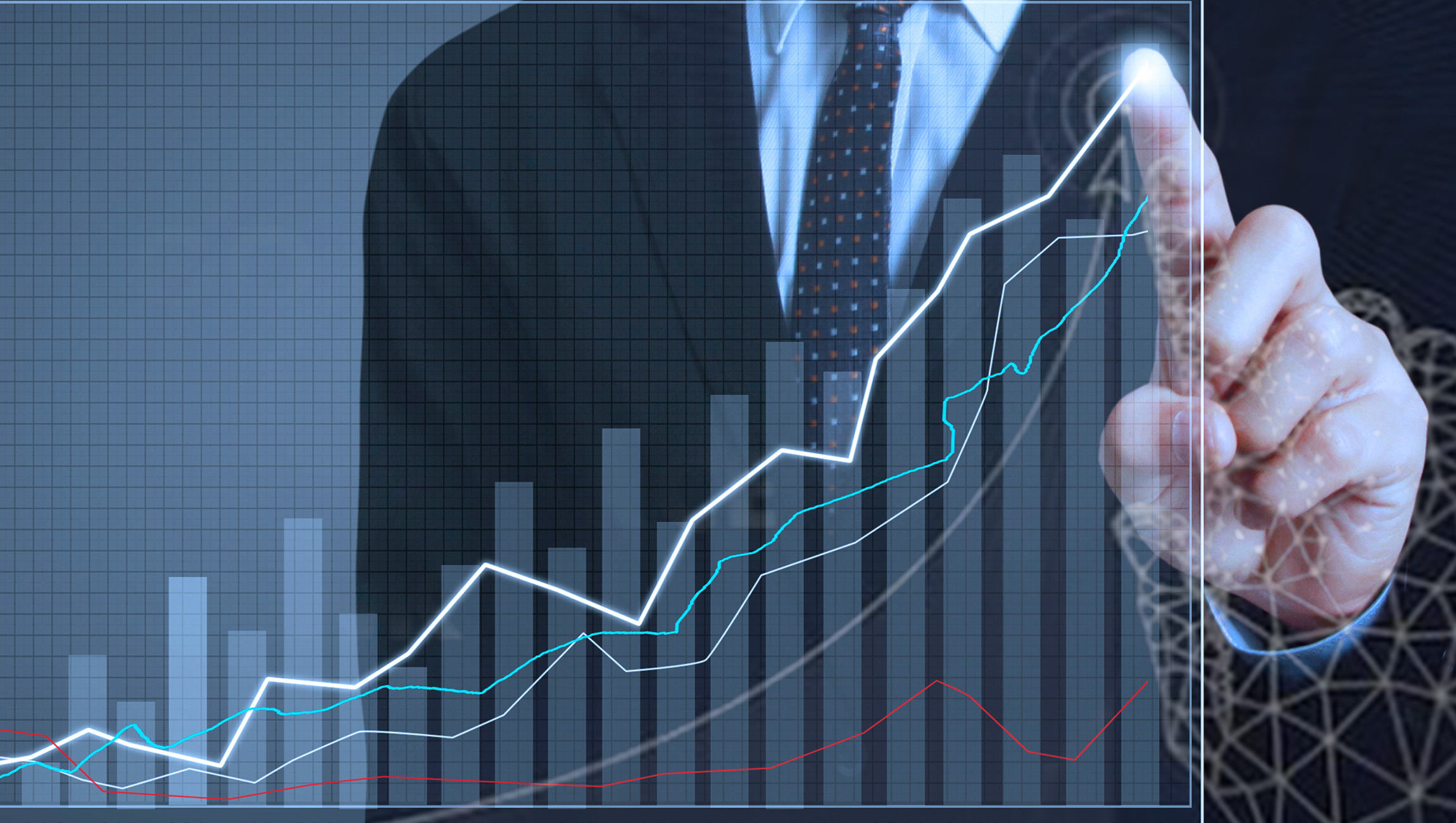
These days, it isn’t hard to find a chorus of experts singing the praises of AI. It’s a heady time, and while all the talk and promises are exciting, it may not be time to pop the champagne just yet. The potential of AI needs to rest on a firm foundation. That foundation is a breadth of quality data, and data needs to become a more central part of the AI conversation.
I want to share with you, perhaps the most important passage you will read about AI. It comes from Forrester Research, and it absolutely nails the way forward-thinking companies and data scientists are viewing the future.
According to Forrester, “the number one challenge for AI adopters is quality data,” and predicts that in 2019, “The tables will turn from AI to information architecture (IA) in the majority of firms that have already dabbled in some form of AI, as they’ll quickly realize that their irrational exuberance for AI adoption must be equally met with solid efforts on an AI-worthy data environment.”
Read More: 605 Expands Executive Team with Strategic Additions to C-Suite and Product Management
Read that paragraph again.
Data, by its nature, is fluid. What’s true today is a snapshot of current conditions that won’t be true tomorrow. And yesterday’s information? Practically worthless – unless you need a dataset for testing purposes or have one that is immune to change. Data degrades, that’s simply part of its nature.
I have spent much of my career thinking about the challenges and potential of data – particularly for sales and marketing professionals. Our company supplies data to help sales and marketers make connections and close deals. Over time, each bit of information a salesperson has on a contact or company will erode. Each of those seemingly small changes (increased revenue, more employees, a phone number change, an email address, a title change) is critical. The longer outdated data feeds an AI system, the less efficient that system will become.
For example, here’s what that happened to us two months ago when DiscoverOrg acquired ZoomInfo. We combined all of the data from both of our systems on customers, particularly data on size, industry, and revenue of our existing customer base to best understand the concentration we had across size and industry spectrums. Initially, using the data that had been sitting in our CRM systems told us the wrong story: that there was a great deal of overlap between the DiscoverOrg and ZoomInfo data. We were hoping that we were going to market against diverse customer segments, and our first pass of the data told us a different story.
So we decided to take a different approach. We took the data, ran it against our APIs that append company firmographics and other attributes. Following this analysis, we found a more accurate story. Why? Because the data that lived in each of our CRM systems were not maintained. Instead, it was appended by legacy vendors historically (here’s looking at you Dun & Bradstreet) and gave us unreliable information. Imagine the types of decisions we were about to make based on this: territory planning, investment in sales teams, market segmentation, marketing spend against specific companies and industries and sizes. These are decisions that will make or break our company over the next three years, and without DiscoverOrg’s API we would have made those decisions against the WRONG insights.
And, guess what, you’re doing this RIGHT NOW. You may have convinced yourself at some point that the data at your disposal isn’t that bad, that it doesn’t make too much of a difference, that you just appended the data, that the sales team is filling it in accurately. STOP. Just STOP. This doesn’t work, and it will hurt you. And picture plugging this flawed data into a machine learning or AI platform and instructing it to “learn” from it. The result: It will lack smarts.
What I’m saying is that sometimes you need to take a step back to question your assumptions when it comes to data and AI. What you do is too important to trust to poor AI recommendations based on bad data. For sales teams who live and die by the quality of their contact data, all the AI suggestions, cadence recommendations, or social selling schedules on the planet are worthless if they don’t have the right data or an actual prospect who is considering a purchase. Without that data, the highest hopes and projections for AI will come crashing down to earth. Not a pretty picture.
Read More: Next Generation of Infor CloudSuite CRM Now Available
None of this is to say that AI doesn’t have a future in sales – far from it.
AI’s ability to identify patterns of behavior can help realize all sorts of business goals. The secret ingredient is the data. It’s what allows one to know with a high degree of confidence which customers should receive another nudge or how long to wait before sending a reminder. It also makes it possible to look at customer response metrics and make more accurate monthly or quarterly forecasts.
The logic underlying this type of analysis would be virtually impossible without the power of AI – and that AI, in turn, would be virtually impossible without a foundation of accurate and timely data. With that data in hand, it’s possible to build customer engagement systems based on what AI can reveal. It’s powerful stuff.
The promise for combining AI and data is real and should be enticing. We first need to understand the business issues, however, and establish data architecture best practices to move forward successfully.
Read More: Vimeo To Acquire Magisto To Power Video Creation For Any Business