AI-Powered Lead Qualification: the Transition from Marketing Qualified Leads (MQLs) to Sales Qualified Leads (SQLs)
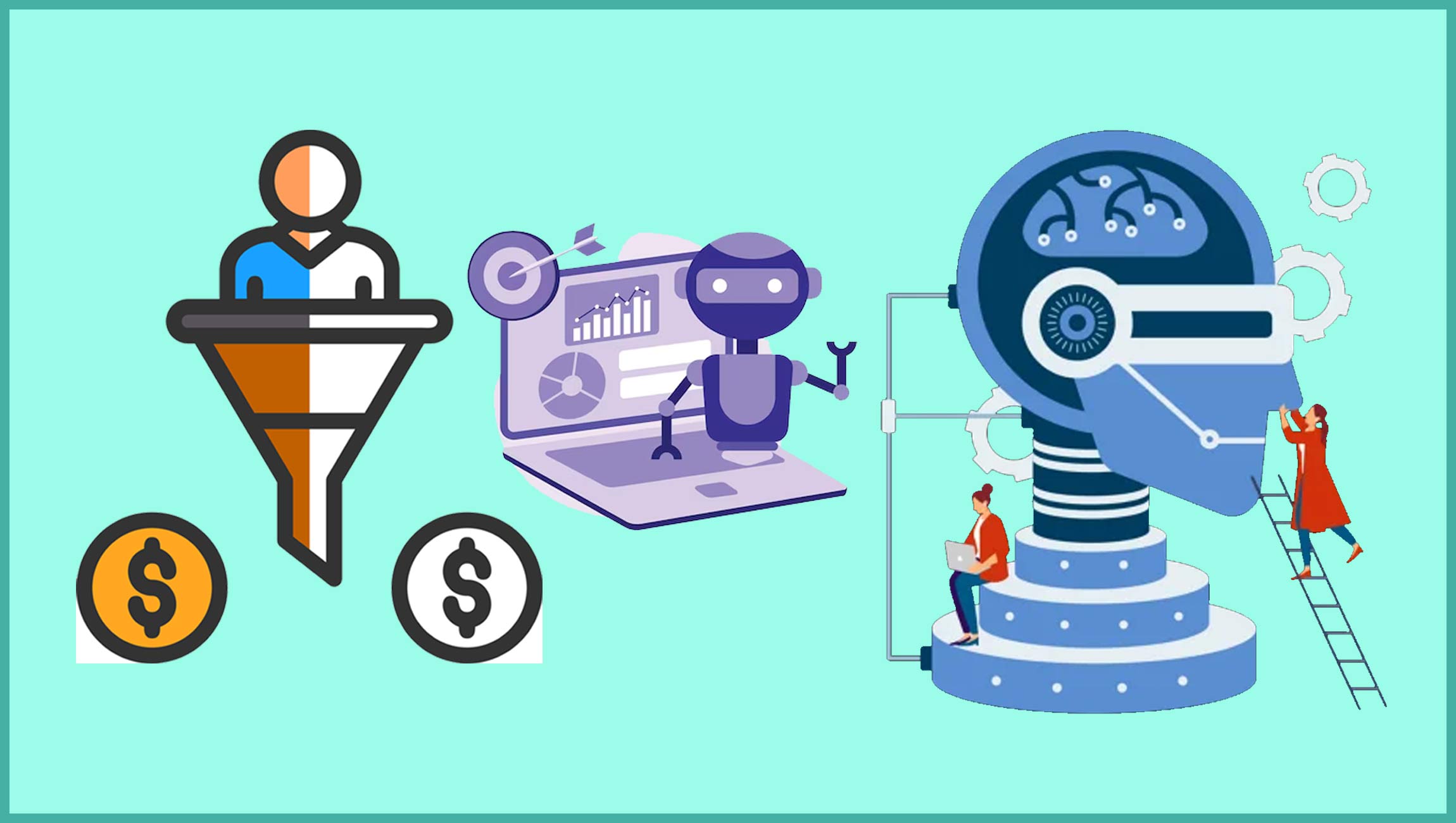
Let’s explore the benefits of Automating the Transition from Marketing Qualified Leads (MQLs) to Sales Qualified Leads (SQLs)
Continue reading to examine how AI-driven automation refines the process of lead qualification as they move through the marketing and sales funnel, helping both Marketing and Sales teams target the right prospects with precision.
AI powered salestech can help forecast your ideal customers and engage them in a smooth, natural format, around-the-clock. That is AI’s lead generation and lead qualification power too. Massive volumes of data are analyzed by AI to find potential customers, and it can accurately forecast who is most likely to be interested in what you offer.
It saves time and frees up your team to concentrate on closing business and developing relationships by automating monotonous chores like email outreach. As opposed to traditional methods, artificial intelligence (AI) can produce leads that are up to three times more qualified while saving up to 50% of your time. Efficiency is critical in the quick-paced field of sales and marketing. However, a startling number shows that, as a result of inadequate lead screening procedures, almost 70% of leads produced by marketing campaigns never become sales.
This inefficiency frequently results from antiquated manual procedures that misalign sales and marketing initiatives, irritating both sides! Making the switch from Marketing Qualified Leads (MQLs) to Sales Qualified Leads (SQLs) is still a major challenge for companies trying to maximize their sales funnels. Errors affect traditional lead qualification processes frequently, wasting money and missing out on chances.
More Insights from The SalesStar Podcast by SalesTechStar
Episode 209: B2B SaaS Marketing and MarTech Best Practices with Ryan Nelsen, CMO at StackAdapt
Though marketing teams work hard to generate leads, many of these leads remain unqualified and stagnate in the sales funnel. As a result, sales teams are forced to sort through unqualified leads, which can be annoying and this eventually lowers conversion rates. Artificial Intelligence swiftly sorts through vast amounts of data and classifies prospective leads. The capacity to cut lead identification time by as much as 70% frees up important resources that can be used for other strategic activities, such as building rapport with those leads.
Businesses can concentrate on what matters creating relationships and closing deals because AI will take care of the heavy lifting. Furthermore, AI goes above and beyond by providing tailored recommendations based on user interests and previous exchanges. This raises the possibility of conversion by enabling firms to approach leads with solutions catered to their unique requirements.
Investigating how AI-driven automation may transform the lead qualification process to address these issues is essential. Organizations can improve coordination between their sales and marketing teams and guarantee that only the most promising leads advance through the sales funnel by streamlining the switch from MQLs to SQLs.
Understanding MQLs and SQLs
Understanding the difference between MQLs and SQLs is essential to understanding the importance of lead qualification. Individuals or businesses who have expressed interest in a company’s goods or services through various engagement indicators, like website visits, content downloads, or email exchanges, are known as marketing qualified leads, or MQLs. Because they have shown some curiosity but have not yet expressed a desire to make a purchase, these leads are regarded as “warm” leads. More nurturing and interaction are usually needed with MQLs to determine their intent and chance of conversion.
Sales Qualified Leads (SQLs), on the other hand, are leads that have undergone screening and are considered prepared for direct sales interaction. These potential customers have shown a greater degree of interest and intent, which is frequently shown by particular actions like asking for a demo, conversing with salespeople, or stating that they need the good or service. When leads are prepared for focused sales outreach, the switch from MQL to SQL is a crucial stage in the sales process.
The Challenge When It Comes To Transitioning Leads Between Marketing and Sales
Even with the explicit definitions, many businesses find it extremely difficult to convert MQLs to SQLs. The misalignment of the sales and marketing teams is one of the primary issues. Marketing teams frequently prioritize producing a large number of leads without getting a clear idea of what, from a sales standpoint, qualifies as a quality lead. The misalignment leads to the transfer of leads that might not be suitable for the sales team’s productive engagement.
One more difficulty is that lead qualification is still done manually. Leads are frequently sent to sales teams based on surface engagement indicators, which can result in wasting time and money on leads that don’t show the required intent to convert. Studies reveal that as many as 50% of leads sent to sales teams are not prepared for a sales interaction, which causes inefficiency and dissatisfaction for both parties.
Furthermore, evaluating leads manually can be laborious and prone to human mistakes. Salespeople may misunderstand engagement data or ignore important signals, which could lead to missed opportunities or improper resource allocation. Not only does this inefficiency have an impact on conversion rates, but it also lowers the spirits of sales teams who are always up against an overwhelming amount of unqualified leads.
A more streamlined and data-driven approach to lead qualification is needed now more than ever as businesses continue to adjust to shifting market dynamics and customer expectations. This is where automation powered by AI becomes useful. Through the utilization of sophisticated algorithms and machine learning capabilities, entities can enhance their lead-qualification procedures, guaranteeing a more seamless conversion from MQLs to SQLs. With AI’s ability to analyze massive volumes of data, spot trends, and forecast lead behavior, marketing, and sales teams can work together more efficiently and precisely to target the correct prospects.
There are considerable but achievable challenges related to manual lead qualification and the switch from MQLs to SQLs. Businesses may overcome these obstacles and establish a more effective and efficient lead qualification process by embracing AI-driven automation. This promotes better conversion rates, which in turn results in higher income and business expansion. It also improves coordination between the marketing and sales teams. We’ll look more closely at the use of AI in lead qualification and examine the different approaches and plans that businesses can use to move leads through the sales funnel as successfully as possible.
The Role of AI in Lead Qualification
In the data-driven corporate environment of today, optimizing sales efficiency and revenue production requires effective lead qualification. Due to manual analysis and subjective judgments, traditional lead qualification processes frequently fail. Artificial intelligence (AI), on the other hand, is completely changing this field by providing strong tools that improve lead qualification via sophisticated data analysis, predictive analytics, and smooth automation. Here’s a closer look at AI’s function in this crucial procedure.
AI-Powered Data Analysis
The ability of artificial intelligence (AI) to quickly and reliably evaluate enormous volumes of consumer data is one of its most important advantages in lead qualification. Conventional approaches frequently depend on a small number of measurements or arbitrary analyses of consumer behavior. On the other hand, a wide variety of data points, such as interactions with websites, social media activity, email responses, and demographic data, can be processed by AI algorithms.
Artificial Intelligence (AI) can identify hidden patterns and trends in this data by utilizing machine learning. AI, for example, may recognize patterns in the behaviors of high-converting leads, including particular website actions or interactions with specific content categories. Businesses can make better decisions by having a deeper understanding of what qualifies as a lead thanks to this thorough study.
Furthermore, AI can continuously improve its analysis in response to fresh data inputs, guaranteeing that lead qualification standards adjust in response to shifts in consumer behavior. This flexibility is essential in a market that is changing quickly, where consumer tastes and habits might change dramatically.
Predictive Analytics for Lead Scoring
Artificial intelligence (AI)-driven predictive analytics greatly improve lead scoring systems by building on the knowledge obtained from data analysis. With the use of statistical algorithms and historical data, predictive analytics makes predictions, allowing marketing and sales teams to focus their efforts on the prospects who are most likely to convert.
AI, for instance, is capable of analyzing previous conversion data to pinpoint the crucial elements that contributed to profitable sales in the past. Predictive analytics can use this information to score leads according to how likely they are to convert.
High-scoring leads can be classified as Sales Qualified Leads (SQLs), and low-scoring leads can be further developed into Marketing Qualified Leads (MQLs). This method accelerates the sales process while also increasing the accuracy of lead scoring. Sales teams can increase their efficiency and effectiveness by concentrating their resources on leads with a higher chance of conversion.
Automating the Transition from MQL to SQL
Finding the appropriate leads to forward to sales is one of the most common obstacles in the migration from MQL to SQL. Through its ability to identify critical actions and engagement cues that signal a lead’s readiness to buy, AI plays a critical part in this process.
AI systems can identify whether a lead is exhibiting signs of interest by analyzing engagement data like email open rates, website visits, content downloads, and social media interactions. A lead may be more prepared to speak with a salesperson if they routinely interact with demo content or browse price pages, for example. Businesses may guarantee that only the most qualified leads proceed forward in the sales process by using AI to track these indications.
Automation Benefits in the Handoff Between Marketing and Sales
Automating the handoff between the marketing and sales teams is a crucial feature of AI in lead qualification. Artificial intelligence (AI) systems that transfer qualification leads automatically based on preset criteria can expedite this procedure.
The solution may instantly alert the sales team and furnish them with pertinent details regarding the lead’s engagement history and interactions when a lead is recognized as an SQL through AI-driven analysis. This guarantees that sales agents have the background knowledge necessary to interact with customers efficiently, cutting down on follow-up time and increasing conversion rates.
Furthermore, automation reduces the possibility of human error, guaranteeing that leads are neither missed nor incorrectly qualified. This smooth transition creates a unified approach to lead management that improves overall productivity by fostering collaboration between the marketing and sales teams.
Benefits of AI-Driven Lead Qualification
The process by which organizations locate, assess, and interact with potential clients has been completely transformed by the integration of Artificial Intelligence (AI) into lead qualification. Comparing AI-driven lead qualification to conventional techniques, which frequently depend on human procedures and static lead-scoring models, reveals many advantages.
Businesses can now increase accuracy, speed, and efficiency throughout the marketing and sales funnel by utilizing real-time data and sophisticated algorithms thanks to artificial intelligence. Let’s examine the main advantages of AI-driven lead qualification, namely as they relate to better accuracy, quicker lead qualification, and more effective sales.
a) Lightning-Fast Insights
The capacity of artificial intelligence (AI) to digest enormous volumes of data quickly and generate insights that would take human teams hours or even days to obtain is one of its greatest advantages. Lead generation technologies that leverage artificial intelligence (AI) can precisely identify potential leads by examining internet activity, engagement patterns, and customer behavior.
AI can detect quality leads up to 50% faster than traditional methods. Businesses may contact prospects before their rivals thanks to this quickness, which gives them a competitive advantage.
b) Improved Precision: Targeting the Right Prospects
The days of firms casting a wide net in the hopes of finding some interested leads are long gone. AI is great at using consumer data analysis to build comprehensive profiles of your ideal leads. AI identifies prospects who are most likely to convert by analyzing their activities, demographics, and preferences.
Businesses that utilize AI for lead generation have seen an improvement in conversion rates of 10-15%, according to a study, because AI makes sure that marketing efforts are directed toward the appropriate audience at the appropriate moment. The capacity of AI to precisely target the correct prospects is one of the most important benefits of lead qualification.
With traditional tactics, marketing efforts may cast a wide net in the hopes of capturing a few promising leads, resulting in a broader and less concentrated strategy. Nevertheless, this may lead to a significant amount of mismatched or unqualified leads being sent to sales teams, which would result in inefficiencies and resource waste. By evaluating enormous volumes of data from many sources, including online interactions, engagement metrics, and customer behavior, AI revolutionizes this process.
Patterns and insights that are not immediately obvious through manual analysis are identified by AI systems. AI, for instance, is capable of identifying minute clues about the intentions of customers, such as regular visits to product pages, social media activity, or email campaign interactions. Artificial intelligence (AI) helps firms find and target leads that have a higher conversion rate by concentrating on these high-value signals.
Furthermore, AI-driven solutions continuously improve their lead-scoring models using data from real-time interactions, so the system learns over time. Because fewer unqualified leads are reaching the sales team, companies utilizing AI for lead qualification claim increased precision. Sales teams can concentrate their efforts on prospects who genuinely express interest thanks to this improved targeting, which raises conversion rates and efficiency levels overall.
c) Personalized Engagement
AI lets companies engage with leads personally in addition to identifying them. AI customizes outreach tactics for optimal effect by examining behavior patterns and preferences.
AI makes sure that each lead feels as though the message is tailored just for them, whether that is done by sending personalized emails or changing the messaging in response to in-the-moment interactions. Engaging leads with personalization makes them feel important, which significantly raises the chance that they will convert.
d) Quicker Lead qualification: Hastening the Procedure
In the quick-paced world of sales and marketing, quickness is essential. Follow-ups with qualified prospects are frequently delayed in traditional lead-qualification processes because of the time-consuming nature of manually sifting through data and evaluating possible leads. Missed chances may arise from these delays, particularly if rivals respond to possible leads more quickly.
This problem is addressed by AI-driven lead qualification, which significantly cuts down on the time required to find and qualify leads by automating the entire process. Large datasets may be analyzed in a matter of seconds by AI, which scores leads according to conversion likelihood and evaluates each one according to predefined criteria.
By removing bottlenecks brought on by human data processing, this automation guarantees that the most promising prospects are promptly identified and forwarded to the sales team for follow-up. AI makes it possible for marketing and sales teams to qualify leads faster and with greater efficiency. Leads that satisfy predetermined standards are automatically identified and forwarded to the relevant salespeople, eliminating the need for laborious departmental handovers or back-and-forth correspondence. Faster response times are a result of this streamlined procedure, and in certain industries, timing may make or break a contract.
AI-driven lead qualification also makes sure that no promising lead is overlooked. Businesses can seize opportunities even after regular business hours thanks to automated systems that can operate continuously, processing fresh data and updating lead scores in real time. Sales teams can interact with qualified prospects more quickly as a result, increasing the likelihood that deals will be closed.
e) Predictive Insights
The capacity of AI to forecast patterns and behaviors is one of its most potent characteristics. AI can predict which leads are most likely to convert by examining historical exchanges and market data. By using predictive lead scoring, companies can focus their efforts on the most valuable prospects first. Sales teams that use AI for lead scoring can see a 30% boost in revenue as a result of spending more time interacting with ready-to-buy leads.
AI is revolutionizing the way businesses find and convert leads by combining lightning-fast insights, improved targeting, personalized interaction, and predictive analytics. Adopting AI is now a must for controlling the market and generating extraordinary growth, not just a choice for remaining competitive.
f) Increased Sales Efficiency: Better Collaboration and Higher Conversion Rates
AI-driven lead qualification improves accuracy and speed while promoting improved teamwork between the sales and marketing departments. In the past, there has frequently been a rift between these two divisions, with marketing teams sending leads to sales teams that might not be completely qualified or prepared for interaction. Reduced conversion rates and inefficiency may result from this imbalance.
Businesses can improve lead quality and departmental coordination by utilizing AI to qualify leads and ensuring that only the most sales-ready leads are forwarded to the sales team. AI technologies offer a comprehensive picture of each lead’s journey by tracking and analyzing customer involvement across a variety of touchpoints, including email marketing, social media interactions, and website visits. AI can ascertain when a lead is prepared to change from a Marketing Qualified Lead (MQL) to a Sales Qualified Lead (SQL) based on this data.
This degree of accuracy in locating qualified leads facilitates communication between sales and marketing. The leads that sales teams receive can be trusted to be truly engaged and prepared to engage, which facilitates easier handoffs and improves departmental alignment. Teams can concentrate on closing agreements with high-potential prospects when fewer unqualified leads are squandering the time of sales representatives, which will ultimately improve the process’s overall efficiency.
Moreover, marketing teams may refine their campaigns based on data-driven outcomes thanks to AI’s insightful insights into the marketing tactics that produce qualified leads the best. This ongoing feedback loop improves sales and marketing coordination and collaboration, resulting in a more unified and successful lead generation and conversion strategy.
g) Increased ROI and Conversion Rates
Higher conversion rates are a natural result of increased accuracy, quicker qualification, and enhanced teamwork. Closing deals is far more likely when AI tools make sure that only the best-qualified leads are sent to sales. Sales teams do not need to waste time on unqualified prospects; instead, they can concentrate their efforts on developing relationships with high-quality leads.
Businesses can also more efficiently organize their resources thanks to AI-driven insights. Businesses may optimize their expenditure and increase return on investment (ROI) by knowing which leads are most likely to convert and which marketing initiatives are producing the best results. This data-driven strategy guarantees more effective use of every marketing dollar while also improving lead creation and qualification.
How to Make a Checklist for Lead Qualification?
To focus on the most promising prospects and streamline the sales process, you must create a lead qualification checklist. It aids in the assessment and classification of every lead in your sales funnel according to particular standards, directing your outreach and engagement tactics.
By using this checklist, the marketing and sales teams may remain productive and in sync, completing more deals with less effort. Now let’s look at how to create a lead-qualification checklist that will help you identify and close more high-quality leads.
a) Consider Their Profile: Identify Who They Are
Any lead qualification process should start with identifying your ideal customer. This entails assessing each lead to determine whether it aligns with your defined buyer personas, which are comprehensive depictions of your ideal clients derived from actual research and data. Demographics such as age, industry, job title, firm size, geography, and pain areas are all included in buyer personas.
Once you are aware of the broad traits of your target market, you can group your leads into other categories, like:
- Decision-makers at the top (such as CEOs or VPs)
- Mid-level supervisors who have purchasing power
- Technical users who are important in the adoption of the product
The idea is to group leads according to these attributes so you can see more clearly where each person belongs in your sales funnel. For example, it is better to deprioritize leads that don’t match the basic requirements, including being in the incorrect industry or location. You can save time by concentrating on leads that fit your target client profile rather than spending it on contacts who are not likely to convert.
b) Evaluate Their Needs Against Your Solutions
The following stage is to determine whether your services or goods address a problem that the lead is concerned with, once you have determined who they are. It’s critical to focus on the prospect’s wants and difficulties in this situation. Address the following questions:
- What are the main issues they are having?
- Which issues are they trying to resolve?
- Are your solutions in line with their priorities as a business?
Your team should now evaluate how your services can meet the unique needs of the potential customer. In your communication, you should highlight the fact that your product addresses a pain issue that a lead may be experiencing, such as streamlining operations.
In the end, you want to be sure that what you have to give and what they require match. When your product is viewed by leads as a business priority rather than merely a nice-to-have, they are more likely to convert. Apply this criterion to weed out leads where there isn’t a strong match and concentrate on those where your offers bring real value.
c) Understand Their Decision-Making Process
Converting a lead isn’t always easy, even if they are a perfect fit for your offering. It is vital to comprehend the decision-making method they use, including the parties involved, the decision-making process, and the schedule.
Consider the following:
- Determine the important decision-makers: Are you conversing with the appropriate individual or are there other parties involved? Occasionally, the individual you are speaking with is not the ultimate decision-maker, but rather an influencer.
- Discover the purchasing procedure: How much time does the company usually take to decide what to buy? Do they need to go through any internal processes first before they can commit?
- Evaluate the purchasing requirements: Which considerations matter most to those who make decisions? Is it cost, features, customer support, or something else?
You may plan how to address the complete buying group by using these questions, which will help you make sure that everyone understands your messaging. This makes the sales cycle move more smoothly and quickly by assisting you in anticipating any demands or objections that might come up throughout the negotiation process.
d) Examine Yourself in the Buyer’s View of the Competition
Knowing where you stand in the eyes of the buyer relative to your competition is a critical component of qualification leads. Directly inquire about your leads’ familiarity with your business and opinions on the competitors. This provides you with important information about how customers view your offerings and what qualities they look for in a provider.
Here’s how to make the most of this knowledge:
- Determine your major differentiators. This will become a crucial talking point in your communications if your prospect appreciates the features or services that you provide but your rivals don’t.
- Take care of any alleged shortcomings: Should a potential customer indicate that they favor a rival’s goods in specific domains, you possess the chance to either fight with your advantages or modify your product’s positioning correspondingly.
- Tailor your messaging: Create customized messaging that draws attention to your advantages while addressing any worries or inclinations they may have regarding rivals. For example, highlight your unique value proposition if customers prefer your price strategy yet find value in your competitor’s customer service.
By being aware of your competitive position, you can improve your pitch and present your solution as the best option for prospects who are still unsure.
e) Evaluate the Budget and Timing
The two most important factors in lead qualification are timing and budget. A prospect may not be prepared to buy even if they are a fantastic fit and adore your service because of financial or schedule restrictions within their company. These are the inquiries you ought to make:
Is the time appropriate? Is this potential customer actively seeking a resolution, or are they merely conducting research? People who are prepared to purchase now or soon should be your main emphasis.
What is the amount of their budget? Do they have the funds to pay for your solution, even if they are interested in it? If not, you might want to put them on a lower-priority list until their economic condition improves. You can make sure that the leads you invest in with your solution are prepared and able to do so by knowing the timing and budget.
f) Nurture Leads That Aren’t Ready Yet
Even if not every lead will be ready to convert right away, that doesn’t mean they should be ignored. Having a nurturing approach is essential for leads who are a good fit but aren’t quite ready to commit. This could entail:
- Regular follow-ups with relevant content
- Adding value via thought leadership (e.g., webinars, case studies, and white papers)
- sending tailored offers as they get closer to decision-making turning points
Taking care of these leads makes sure that your business comes to mind when they’re ready to make a purchase. Much of this nurturing may be completed automatically with the use of AI and marketing automation, resulting in tailored interaction with less need for significant manual labor.
Your sales and marketing teams will remain in sync when it comes to finding and pursuing high-potential prospects if you have clear lead qualification criteria in place. Your lead qualification process can be streamlined by concentrating on the appropriate profiles, assessing demands, comprehending decision-making procedures, and taking the competition into account. This increases productivity, boosts sales, and propels business expansion by enabling your sales staff to concentrate their efforts on leads that have the highest conversion rates.
Best Practices for Implementing AI in Lead Qualification
Artificial intelligence (AI) has revolutionized lead qualification processes, changing how firms prioritize and manage prospective clients. Businesses can streamline their marketing and sales efforts to concentrate on the most promising leads by utilizing AI’s capacity to evaluate massive volumes of data and forecast consequences.
However, companies need to follow a set of best practices if they want to fully realize the promise of AI in lead qualification. Two crucial areas that stand out as crucial tactics for guaranteeing success are constant optimization and data-driven segmentation.
Read More: SalesTechStar Interview with Christina Brady, CEO of Luster AI
How AI-Driven Segmentation Works?
AI analyzes data from a variety of touchpoints, including website visits, email exchanges, social media activity, and CRM entries, by utilizing machine learning algorithms. AI systems can rate leads according to their propensity to convert by analyzing these actions, giving businesses the ability to target the most qualified leads for prompt follow-up.
Additionally, AI systems can spot trends that human analysts would overlook, exposing undiscovered possibilities for outreach.
1. Behavior-Based Segmentation:
AI programs examine how prospects engage with your emails, social media posts, and website. A lead who often interacts with product demos or visits your price page, for instance, is probably more interested in purchasing a lead who merely reads your blog entries.
AI ensures that the sales team concentrates on leads who have demonstrated true buying intent by classifying leads based on these characteristics.
AI is also capable of segmenting leads based on firmographic (such as industry, and company size) and demographic (such as age, and location) information. This kind of segmentation is particularly helpful for business-to-business (B2B) transactions, as it helps to create a customized sales approach by providing insight into the requirements of various industries or sectors.
2. Interaction-based Segmentation:
AI systems divide up leads according to their degree of interaction with your brand, in addition to examining their actions. Highly engaged leads are those who actively download white papers, attend webinars, and open emails; less-responding prospects could require additional nurturing before they’re ready to convert.
Benefits of AI-Driven Segmentation
AI guarantees that sales and marketing initiatives are aimed at the appropriate target market through precision targeting. Leads in high-conversion categories are more likely to engage with sales teams because they receive messages that are specifically customized to their needs.
1. Enhanced Efficiency:
By giving priority to leads with the highest conversion rates, sales teams may avoid squandering time on unqualified leads. AI segmentation frees up resources so that your team may concentrate on high-value exchanges.
2. Enhanced Personalization:
Businesses may now mass-personalize their outreach thanks to AI. Through targeted content delivery to each category, businesses can strengthen their relationships with leads and boost conversion rates.
Using data-driven segmentation in your lead qualification process is essential to improving accuracy and making sure that your marketing and sales campaigns are productive and successful.
3. Continuous Optimization: Gradually Improving AI Algorithms
Even though lead qualification enabled by AI has many benefits, it is not a one-time fix. For AI to yield long-term benefits, it needs ongoing optimization. Artificial intelligence (AI) algorithms require constant monitoring and improvement to function better, much like a human sales staff requires continuous training and feedback.
Businesses can adjust their AI models to changing market conditions, consumer behavior, and corporate objectives, maintaining the high efficacy of their lead qualification process.
The Significance of Ongoing Optimization
To rate leads and make predictions, AI models rely on past data. Markets are dynamic, though, so what has historically worked might not always translate to the future. New trends appear, industries change, and customer tastes change. AI models can become out of date without regular updates, which can result in erroneous lead scoring and inadequate segmentation.
For example, the AI system needs to be retrained to account for changes made by the company, such as introducing a new product or targeting a different market group. Similarly, the AI model needs to modify its criteria to account for new realities if external variables (such as new competitors, economic upheavals, or technology improvements) cause changes in customer behavior.
Best Practices for Ongoing Optimization
The following are the best practices for continuous optimization:
1. Frequent audits of data:
The caliber of the data your AI model consumes will directly affect the caliber of the model’s output. Make sure your data sources are reliable, current, and relevant by regularly auditing them. Remove duplicates, out-of-date facts, and inconsistencies from your data to avoid skewing AI forecasts.
2. Feedback Loops:
Put in place feedback loops that let your team offer suggestions on how the AI model is performing. For example, the sales team can mark cases for additional investigation if they observe that leads with high scores are not converting as anticipated. These comments aid in improving the AI’s scoring system.
3. Retraining AI Models:
To make sure your AI models remain current, retrain them whenever new data becomes available. Retraining could be required every few months or following major changes to your firm, such as new product launches or changes in customer behavior. Retraining enables AI to stay predictively accurate while adjusting to new patterns.
4. A/B Testing:
Test various AI models and lead qualification strategies on a regular basis. To determine which combinations produce the best results, you can test out various scoring criteria, engagement metrics, or behavior-based groups. You can optimize the effectiveness of your AI strategy by comparing results from A/B testing.
5. Track Performance Metrics:
To measure the effectiveness of your AI lead qualification efforts, set up key performance indicators (KPIs). Lead-to-conversion rates, average deal size, and time to close are a few examples of metrics that can show how well the AI is working and where it can be improved. Examine these indicators often to identify patterns and potential areas for improvement.
Benefits of Ongoing Optimization
The following are the benefits of continuous optimization:
1. Adaptability:
AI models that are continuously adjusted have the ability to adjust to shifts in consumer behavior and market dynamics, guaranteeing that your lead qualification procedure is still applicable and efficient.
2. Increased Accuracy:
As AI algorithms are improved over time, more qualified leads and higher conversion rates result from their predictions and lead scoring.
3. Long-Term Competitive Advantage:
You can outperform rivals who might be employing antiquated or stagnant systems by continuously refining your AI-driven procedures. AI can be utilized as a long-term, strategic asset with continuous optimization.
The effectiveness of integrating AI in lead qualification with your sales and marketing processes depends on adhering to best practices. Leads are correctly classified according to their behaviors and levels of engagement when you concentrate on data-driven segmentation. In the meantime, ongoing optimization enables you to adjust to evolving circumstances, guaranteeing that your AI model maintains its high level of efficacy over time.
When combined, these tactics will enable you to fully utilize AI for lead qualification, which will improve targeting, increase conversion rates, and foster long-term business expansion.
Best Practices for Implementing AI in Lead Qualification
The way businesses find and prioritize high-quality leads is fast changing due to AI-powered lead qualification. Businesses can increase efficiency, accuracy, and conversion rates by utilizing cutting-edge technology like machine learning, natural language processing (NLP), and data analytics in their lead qualification process. Let’s examine some actual cases from a variety of industries to demonstrate how artificial intelligence is transforming lead qualification.
1. B2B Company Enhances Sales Pipeline
The problem for B2B software provider Acme Solutions was sorting through a big lead pool and selecting high-value prospects. They used to have a laborious and inaccurate manual lead certification process. To solve this, Acme Solutions put in place an artificial intelligence (AI) driven lead qualification system that examined important information such as website activity, industry vertical, interaction history, and organization size.
Based on trends found in past customer data, the AI system employed machine learning algorithms to forecast which leads were most likely to convert. The sales team was able to concentrate their efforts on the prospects that had the greatest potential for conversion because of these insights.
Results:
The result was a 15% boost in overall conversion rates and a 30% increase in Acme Solutions’ sales pipeline. Their sales force was able to allocate resources more efficiently and increase revenue growth by spending less time on unqualified leads thanks to the automatic prioritization of high-value prospects.
The following illustration shows how AI may deliver precise, data-driven insights that result in more successful sales strategies, enhancing productivity and results.
2. AI-Powered Chatbot Identifies Website Visitors for Online Sales
The e-commerce store Bloom & Co. needed to engage with visitors and qualify prospects in real-time, but they couldn’t handle every request by hand. They employed an AI-powered chatbot that utilized natural language processing (NLP) to converse with users and ascertain their needs to address this.
To find out about visitors’ interests, preferences, and desire to buy, the chatbot asked certain queries. After that, it assessed the answers to ascertain the chance of conversion. The proper sales representative received qualification leads based on this data, guaranteeing that the relevant prospects were given priority for follow-up.
Results:
The AI chatbot increased the accuracy of lead qualification and gave clients a seamless, conversational experience. By giving personalized answers to each visitor’s demands, this enhanced conversion rates in addition to visitor engagement. Bloom & Co. had a notable increase in conversions and a more effective sales procedure.
This example shows how well-implemented AI chatbots can manage enormous amounts of client inquiries and offer customized lead qualification, enhancing customer satisfaction and sales efficiency.
3. AI Lead Scoring Helps Provide High-Value Priority
Multinational tech corporation Global Tech Inc. found it difficult to set priorities for the vast amount of leads it was receiving. Their sales team was overburdened with the task of deciding which of the thousands of new leads produced by marketing efforts to concentrate on.
An AI lead scoring algorithm was put into place by Global Tech Inc. to evaluate a variety of data elements, including lead source, website behavior, industry, and demographic data. Based on these variables, the AI system ranked the leads according to their chance of conversion and assigned a score to each one. Lower-scoring prospects were put in nurturing workflows for additional development, while the highest-scoring leads were automatically moved to the top of the sales team’s priority list.
Results:
Global Tech Inc. saw a 20% improvement in sales win rate and a 40% reduction in lead qualification time thanks to the AI model. The organization increased sales efficiency and witnessed faster transaction closure times by concentrating on the most promising prospects.
This example shows how AI may be used to analyze large, complicated datasets and prioritize leads, allowing businesses to concentrate their efforts where they have the most chance of success.
4. Lead Qualification Time Is Cut Down by a Marketing Agency
Rapidly expanding digital marketing firm Velocity Marketing encountered issues with their manual lead qualification procedure. Their sales staff frequently missed opportunities and put off follow-ups because they spent hours evaluating and qualification prospects. Velocity Marketing added an AI-powered lead qualification system to their CRM to solve these inefficiencies.
The AI algorithm swiftly identified which leads matched the agency’s ideal client profile by analyzing vital lead data, like job title, firm size, industry, and budget. The technology qualified leads in a matter of minutes by automating the preliminary lead screening process, which relieved the sales team of some of their manual labor.
Results:
Velocity Marketing achieved a 60% reduction in lead qualification time. Instead of squandering time on manual data evaluation, this allowed the sales staff to concentrate on cultivating relationships with qualified prospects. The agency’s sales productivity increased noticeably as a result, closing more agreements in less time.
This example demonstrates how artificial intelligence (AI) may expedite laborious procedures, resulting in quicker and more effective lead qualification and freeing up teams to concentrate on higher-value tasks.
5. AI Enhances Multi-Channel Lead Qualification for a SaaS Company
Focus Technologies, a SaaS firm, sought to handle multi-channel lead qualification across email marketing, webinars, and website traffic more effectively. They were able to combine data from several channels and track lead engagement comprehensively by implementing an AI-powered platform.
Predictive analytics was used by AI systems to automatically evaluate behavior across all touchpoints and determine which prospects were prepared for a sales talk. The sales team was able to give priority to leads who had consistently demonstrated interest and interaction across several platforms thanks to the cross-channel connection.
Results:
Focus Technologies had a 25% improvement in lead quality thanks to the AI platform and an increase in qualified leads from all channels. The organization was able to more clearly detect patterns of engagement thanks to this multi-channel approach, which led to more targeted follow-ups and increased conversion rates.
This example demonstrates how artificial intelligence (AI) may optimize lead qualification across several channels, combining consumer encounters into a single, all-encompassing image that improves sales tactics.
The Ability of AI to Revolutionize Lead Qualification
These actual cases demonstrate how AI is revolutionizing lead qualification in a variety of sectors. Businesses can select high-quality leads with unmatched precision thanks to artificial intelligence (AI), which can automate tedious operations, analyze large data sets, and personalize consumer interactions. Companies may boost their sales pipelines, shorten lead qualification times, and eventually increase conversions and revenue growth by concentrating on the most promising prospects.
Not only is implementing AI-driven lead qualification a competitive advantage, but it’s quickly becoming a need for companies looking to stay ahead of the curve in today’s fast-paced industry and maximize sales and marketing efforts. The potential for innovation and growth in lead management will only increase as more businesses use AI solutions.
Future Trends in AI for Lead Qualification
Businesses are redefining lead qualification in a way that is more data-driven, precise, and efficient as a result of the rapid growth of artificial intelligence (AI). AI technology is opening the door to even more creative ways to find, interact with, and win over new clients as it develops. Advanced machine learning for improved lead scoring and AI’s growing role in real-time lead engagement through conversational AI are two major developments influencing the future of AI in lead qualification.
1. Advanced Machine Learning: Enhancing Lead Scoring and Prediction Accuracy
Many lead qualification systems already use machine learning (ML), a component of artificial intelligence. It’s an effective tool for enhancing lead scoring and conversion prediction because of its capacity to evaluate large volumes of data and identify trends. We might anticipate even bigger improvements in these domains as machine learning models become increasingly complex.
Optimising Lead Scoring Frameworks
Present AI-powered lead scoring models score leads based on a mix of firmographic, behavioral, and demographic information. The kinds of data these models employ or the original guidelines established by sales and marketing departments may still place restrictions on them. Future models will be able to continuously learn and adapt without the need for human involvement thanks to developments in machine learning.
These sophisticated models will
-
Add More Diverse Data Sources:
In the future, lead scoring systems will incorporate data from a wider range of sources, such as competitive behaviors, sentiment analysis, social media interactions, and real-time buyer intent signals. This will produce more accurate evaluations that take into account a lead’s changing requirements and behavior in addition to their current situation.
-
Adapt Over Time:
Machine learning algorithms will continually improve their accuracy with each lead engagement as organizations collect more data from their lead engagements. This will decrease the likelihood of concentrating on low-potential prospects by enabling firms to predict a lead’s desire to purchase with even better accuracy.
-
Better Forecasting Models
In addition to scoring, more sophisticated machine learning models can more accurately forecast a lead’s chance of converting. To do this, it will be necessary to analyze past data in addition to applying predictive analytics to estimate future behavior based on complex variables including evolving buyer preferences, changing market trends, and even more general economic patterns.
For instance, by determining when leads similar to yours have previously converted, machine learning algorithms may be able to forecast the best time to reach out and modify scores in response to price adjustments made by competitors. Hyper-personalized lead qualification methods resulting from this will increase conversion rates and guarantee that the sales force is concentrating on leads with the best chance of succeeding.
2. AI for Real-Time Lead Engagement:
The increasing use of AI in real-time lead interaction is another trend in lead qualification going forward. Natural language processing (NLP) and machine learning-driven conversational AI are transforming the way businesses engage with leads by enabling instantaneous qualification while retaining a human-like touch.
-
Conversational AI for Immediate Interaction
Future AI systems will take lead interaction management to a whole new level. Currently, a lot of firms utilize chatbots or virtual assistants to handle first-lead conversations. Businesses will be able to interact with leads in real-time, collect information, and qualify them with ease by combining conversational AI with more sophisticated machine learning algorithms.
-
Understanding Lead Intent:
As conversational AI advances, it will be able to decipher a prospect’s true intentions in addition to the words they use. This will enable the system to pose more insightful queries and steer the discussion in the direction of learning more about the needs, problems, and decision-making process of the lead.
-
Personalized Real-Time Conversations:
In the future, artificial intelligence systems will have the capacity to draw from a vast dataset of consumer encounters to present prospects with highly tailored dialogues. These technologies can identify each lead’s distinct purchasing path and adapt their responses according to the lead’s previous encounters with your brand or even those of your competitors.
If a prospective customer inquires about a product, for instance, conversational AI may examine their browsing history, gather information from previous exchanges (like email clicks or social media activity), and immediately qualify the lead by posing pertinent follow-up queries. With the capacity to offer customized, real-time discussions, lead qualification times will be greatly shortened, and sales teams will always have access to the most recent data.
-
Proactive Engagement and Real-Time Decision Making
AI will eventually advance from reactive chatbots to proactive lead engagement. AI will track lead behaviors, like as time spent on a pricing page, downloads of whitepapers, or mentions of the business on social media, and use this data to reach out proactively, rather than waiting for the lead to make contact.
For example, AI might start a tailored dialogue with a lead who repeatedly visits a product comparison page, addressing their issues and further qualification them. In addition to expediting the lead qualification process, this proactive AI-driven interaction will foster relationships in a way that seems natural and beneficial to the prospect.
Conversational AI in Multichannel Experiences
AI will eventually make real-time interaction possible across a variety of channels. AI will allow businesses to interact with prospects through voice assistants, chat, email, and social media, smoothly transferring the dialogue between different channels. No matter how a prospect engages, the AI will save context, guaranteeing that the interaction is relevant and customized.
AI systems will combine engagements, such as a lead interacting with your brand through a chatbot on your website and then following up on social media, to keep the relationship flowing smoothly. With a more seamless and customized experience brought about by this real-time, multichannel interaction, businesses will find it simpler to move prospects through the funnel with less assistance from humans.
Businesses will experience significant benefits in lead qualification, prospect engagement, and sales process efficiency as AI develops further. Advanced machine learning will make it possible to score and anticipate leads with extreme accuracy, and conversational AI will revolutionize real-time engagement by enabling companies to qualify leads instantaneously through thoughtful, personalized Interactions.
These developments will improve lead qualification efficiency while also giving prospects a smoother and more customized experience, which will increase conversion rates and boost overall sales success. Companies that use these AI-driven technologies will be able to position themselves as leaders in their respective areas and generate a lot of leads and sales.
How Marketing and Sales teams Can precisely identify suitable prospects Using AI-Driven Automation?
The lead qualification process is being revolutionized by AI-driven automation, which is providing MarTech and SalesTech teams with previously unheard-of accuracy and productivity. AI technology refines every stage of a lead’s journey as it moves through the marketing and sales funnel, making sure that only the most relevant and prospective prospects are identified and given more time to develop.
1. Data-Driven Precision
Businesses can use artificial intelligence (AI) to evaluate massive volumes of data on potential leads and find patterns and insights that human teams might overlook. AI generates comprehensive profiles of prospects by monitoring their online activities and evaluating their firmographic and demographic information. Marketing teams may now more precisely categorize leads, enabling them to send communications that are relevant to the proper audience.
AI, for instance, can discover leads that have the highest potential by analyzing consumer data like as browsing history, frequency of interactions, and content engagement. AI digs deeper insights, such as forecasting purchase intent based on minute behavioral clues, rather than depending just on surface-level metrics. The effectiveness of the lead nurturing process is increased overall because of this exact targeting, which guarantees that marketing initiatives reach leads who are most likely to convert.
2. Lead Scoring with Predictive Analytics
Using predictive analytics for lead scoring is one of the biggest benefits of AI in lead qualification. Marketing and sales teams have historically relied on manual scoring according to predetermined standards such as job title or size of organization. But AI-powered lead scoring goes far further than that; it uses predicted algorithms and historical data to give each lead a score that is much more accurate.
AI can recognize the characteristics of high-quality leads by examining trends from prior successful conversions. Analyzing elements such as the frequency of email contacts, engagement with particular content kinds, or particular behaviors that suggest a desire to purchase could be part of this. Teams can more efficiently prioritize leads with the use of predictive lead scoring, which helps sales teams concentrate their attention on the prospects that have the highest likelihood of closing deals.
3. Simplified Lead Transfer Between Sales and Marketing
A frequent issue that companies face is the seamless transfer of leads from marketing to sales. Premature or improperly qualified leads are frequently ignored, which can result in inefficiencies and wasted effort. This problem is solved by AI-driven automation, which makes sure that only leads that are properly qualified are sent to the sales and marketing teams, improving their cooperation.
By tracking important metrics like lead engagement and purchase readiness, artificial intelligence (AI) technologies can identify when it’s best to convert a lead from marketing qualified lead (MQL) to sales qualified lead (SQL). For instance, AI can indicate that a lead is prepared for sales outreach if the lead has interacted with a particular product page several times, downloaded pertinent materials, or spoken with a company person directly. By doing this, the likelihood of an early engagement is decreased and tension between the two teams is decreased.
4. Personalized Engagement at Scale
Additionally, AI makes it possible for automatic, customized interaction that appeals to specific leads. AI may customize communication depending on a lead’s choices, actions, and previous exchanges by using machine learning algorithms. A more meaningful connection is made with prospects when outreach attempts are thus personalized, increasing the likelihood that they will respond favorably to the efforts.
AI-driven chatbots, for example, can engage in real-time conversation with website visitors to collect information and qualify leads based on their queries. Because these chatbots are always available to engage leads, no chance is lost. AI increases the overall productivity of the lead qualification process by automating the initial phases of engagement, freeing up marketing and salespeople to concentrate on more intricate interactions.
5. Constant Improvement and Learning
As AI systems handle more data, they constantly learn and get better. AI-driven solutions, in contrast to conventional lead qualification techniques, are flexible enough to adjust to changing market conditions and new patterns. AI models improve their capacity to anticipate which leads will convert by refining their procedures as they are exposed to more successful conversions and unsuccessful tries.
Over time, this continual optimization makes sure that companies are using the most recent and correct insights, increasing the effectiveness of their marketing and sales initiatives. AI gains insights from previous campaigns to further refine targeting in the future.
Final Thoughts
The process of qualifying leads has been completely transformed by AI, which has also changed how companies find, interact with, and win over new clients. Artificial intelligence (AI)-powered solutions can swiftly sort through enormous volumes of data using sophisticated machine learning, increasing lead scoring accuracy and cutting down on lead qualification time.
Artificial intelligence (AI) plays a revolutionary role in lead qualification by providing creative solutions. Businesses can optimize their lead-qualification processes and precisely target the right prospects by leveraging AI-driven data analysis, automation, and predictive analytics. Effective lead qualification will become a crucial component of winning sales strategies as companies continue to adopt AI technologies.
This will increase conversion rates and optimize revenue potential. Through more precise prospect targeting and reduced effort waste, businesses can increase the likelihood of closing deals by utilizing these AI-driven technologies. Businesses may boost sales productivity, prioritize leads more effectively, and eventually increase revenue growth with improved predictive analytics.
Finally, other advantages improve the lead-generating process overall with AI-driven lead qualification. Artificial intelligence is revolutionizing the way businesses locate, assess, and interact with potential consumers. It does this by improving prospect targeting precision, accelerating lead qualification, and increasing sales efficiency.
AI helps sales and marketing teams work together more efficiently by streamlining the lead qualification process, which raises conversion rates and improves overall business results. Utilizing AI for lead qualification is not only advantageous in the current competitive scenario, but also essential for companies hoping to prosper and expand.
Read More: The Potential of Artificial Intelligence in Sales Enablement
Conversational AI, another feature of AI that ensures relevant and tailored interactions at every touchpoint, allows for real-time contact with potential customers. AI has simplified the process of accurately and swiftly qualification leads, whether via chatbots or multichannel contact. This has allowed for a smooth transition of leads from marketing to sales teams. Artificial intelligence (AI) streamlines the whole marketing and sales funnel by employing predictive insights and automating repetitive operations, enabling firms to work smarter, not harder.